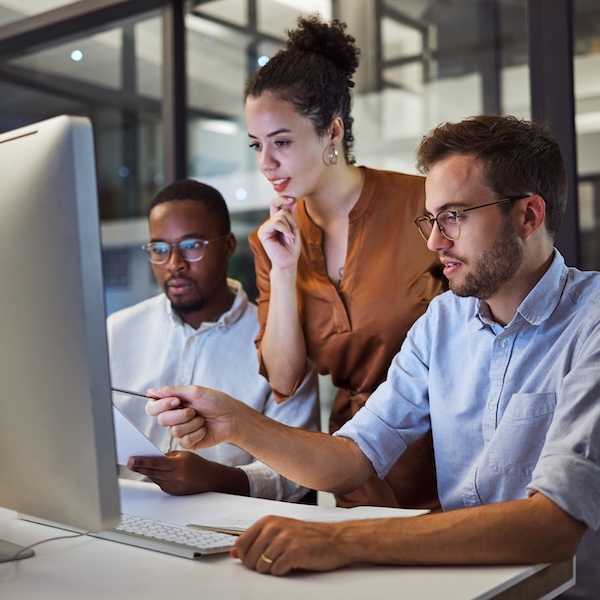
Why do you need QA expertise for AI?
Keep up with the fast pace of AI and changing industry needs
- Compliance standards all are heavy on testing based on what was provided by EU AI Act and Biden administration’s AI Bill of Rights and over 13 states with proposed or enacted legislation.
- QA mission is to reduce risk to company and the community with the delivery of new technology.
- Rapidly changing technology requires best in practice quality standards that ensure focused on how technology is used for meeting end user outcomes.
QualityWorks Driving AI Governance
Here are some specific ways in which QualityWorks drives to AI governance
Setting Standards and Best Practices
QA teams can help define and implement best practices and standards for AI development and deployment. This includes establishing criteria for data quality, model transparency, fairness, and security. By setting these standards, QA helps create a framework within which AI systems must operate, ensuring consistency and compliance across different projects. We use best practices based on regulatory readiness and risk management guidelines like the National Institute of Standards and Technology AI Risk Management Framework.
Regulatory Compliance
QA ensures that AI systems comply with local and international regulations. This includes testing for compliance with privacy laws, accessibility standards, and industry-specific regulations. By doing so, QA helps organizations avoid legal penalties and reputational damage.
Data Quality Evaluation and Continuous Monitoring
Governance is not just about setting rules; it's also about ongoing oversight. QA is integral in monitoring the performance and behavior of AI systems post-deployment to ensure they continue to operate as intended and within ethical and legal boundaries. This involves regular audits, performance evaluations, and the implementation of mechanisms to detect and correct deviations from expected behavior.
Ethical Assurance
QA teams can be tasked with ethical assurance, ensuring that AI systems do not inadvertently harm users or perpetuate biases. This involves ethical audits, where systems are reviewed not just for what they do, but for the broader implications of how they do it.
In essence, QA in AI governance is about embedding quality, safety, and ethical considerations into the fabric of AI development and operation. It ensures that AI technologies not only meet technical specifications but also adhere to broader societal values and legal standards.
Ethical Assurance
AI systems can pose various risks, from technical failures to ethical breaches. QA in AI governance involves identifying potential risks at different stages of the AI lifecycle and implementing strategies to mitigate these risks. This could include stress testing, scenario analysis, and sensitivity testing to understand how changes in data or environment might affect the system.
Our Risk Management platform provides an AI Risk Management framework based on the NIST and leading industry expertise.
Promoting Transparency and Accountability
QA practices in AI governance help in documenting the development process, decision-making criteria, and performance metrics of AI systems. This documentation is crucial for transparency, allowing stakeholders to understand how decisions are made and providing a basis for accountability.
Stakeholder Engagement
Part of AI governance involves engaging with various stakeholders, including users, regulatory bodies, and the public, to understand their concerns and expectations. QA can facilitate this by conducting user testing, gathering feedback, and incorporating it into AI development and policy-making.